How Behavioral Data Can Be Used to Empower Business Decisions
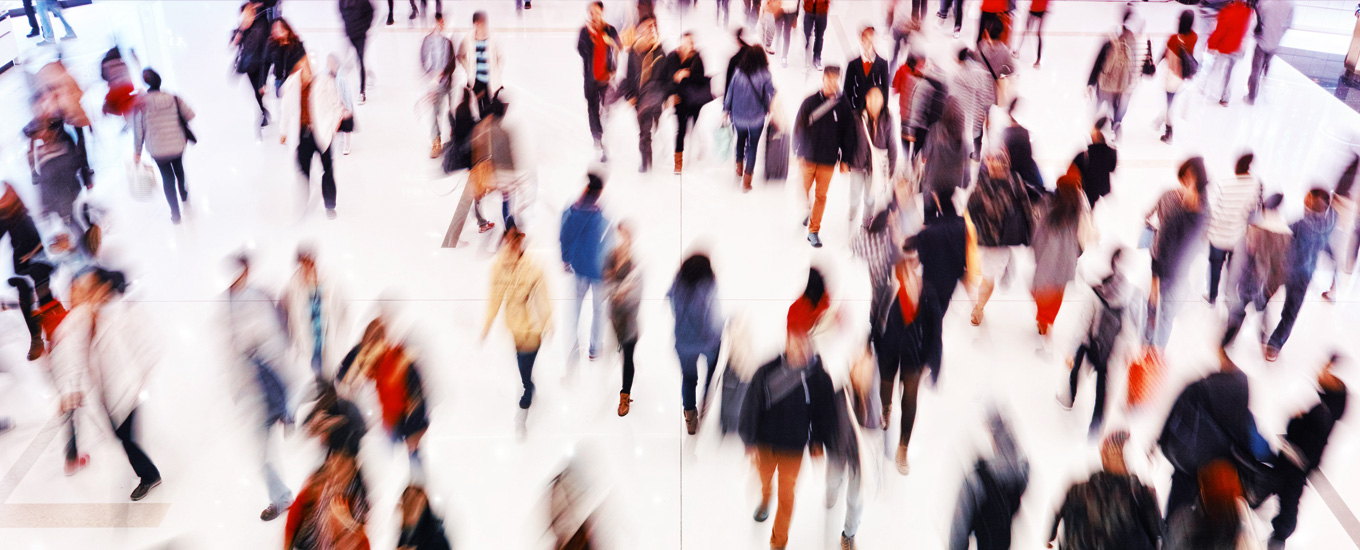
Managing crowds in venues such as stores, airports, and stadiums is a science. Done well, managing the flow of people in public spaces makes life safer and more pleasant for customers and operations more efficient for businesses. Increasingly, behavioral data capture is being used to do so. This growing field requires technology such as computer vision and a diverse team of experts with deep knowledge of consumer behavior and local cultures. Data privacy is also an important aspect, and data collection must always happen in a secure and informed environment ensuring that a mindful approach is adopted.
Behavioral Data Capture Defined
Behavioral data capture is the process of observing human behavior typically in crowded areas such as stores, airports, and casinos. For instance, airports can do a better job knowing when and where to assign extra personnel such as security and customer service personnel by observing where crowds are clustering, especially during peak travel times. Retailers can keep stores safer by observing every corner of the store in order to detect security threats. Casinos can detect attempts at cheating by monitoring guests and employees.
Human beings cannot do all this alone. They need help. This is where behavioral data capture comes into play.
One of the core components of behavioral data capture is computer vision. Computer vision is a form of artificial intelligence (AI) that makes it possible for computers to analyze visual data such as pictures and video and make intelligent decisions based on that analysis in real time. Google uses computer vision to help people perform visual search more effectively. Autonomous vehicles require computer vision to detect objects, lane markings, signs, and traffic signals in order to drive safely. Airports use computer vision to manage security, as we recently shared in a blog post.
Computer vision can capture far more data than any human being could ever hope to observe with the naked eye. And cameras equipped with computer vision can keep a business informed in real time (such as whether lines are getting too long at an entrance to a stadium). By contrast, cameras without computer vision simply record footage that needs to be examined later without the benefit of real-time reporting.
But computer vision only describes what is happening without meaningful interpretation of an event. Computer vision alone cannot capture the myriad and often subtle ways people behave. People with bad intentions, such as shoplifters, are constantly finding new ways to commit crimes, and computer vision may not observe newer criminal scams because it only observes what it has been trained to observe. Nor is computer vision scalable for businesses that have traditionally used only point solutions for very specific applications. Computer vision needs pattern recognition to consistently detect behavior and people to feed new data to the AI behind a computer vision application. Computer vision describes what is happening; pattern recognition interprets what’s happening. Computer vision and pattern recognition together can help a business deliver powerful results.
How a Business Trains Computer Vision
As noted, for behavioral data capture to be effective, AI needs to be trained to know what to look for. For instance, AI must know how to discern the difference between a shopper who is browsing and one who is stealing. It needs to be fueled by annotated/labeled data. The models need to be tested and validated. And in a world with increasingly stringent privacy laws, businesses must proceed carefully. Moreover, training AI gets complicated when a business has a limited inventory of useful data to work with, such as clear and complete sample footage of people in stores committing security breaches.
At Centific, we rely on two approaches for training:
Centific Labs
We operate a simulated retail space that makes it possible to re-create scenarios such as store theft or customers forming long lines that need to be managed with better crowd control. We do this with real people acting in roles assigned to them. From there, we record behaviors and use the footage to train computer vision accordingly.
Typically, we give our test subjects very minimal guidance on the scenario we want to record (shopping, theft, etc.). That’s because we want to record all the ways people behave naturally in a real store. If our trainers provide too much detailed instruction, they limit the behaviors we want to record.
Collection in the Field
We also rely on our OneForma platform to globally source teams of people to help us record scenarios that can be more easily recorded in real life, respecting security requirements, of course.
For example, a client recently asked us to improve its home security camera, specifically by doing a better job notifying residents that packages have been left on their doorsteps. Doing this required us to train cameras to understand all the different ways someone might place a package near a homeowner’s front door. This might sound simple, but it’s not. No two residences are exactly the same. Computer vision needs to account for myriad variables such as dim light, ambient noise, the exact position of a package (e.g., leaning against a door? Slipped beneath a mailbox?), and so on.
To do this, we relied on a globally crowdsourced team to collect data. We asked our team to feed into our Data Collection & Annotation platform actual footage of packages being dropped in front of their personal homes. (We trained them on the technicalities of how to do all this). This crowdsourced approach gave us the data we needed to train AI to really understand what package delivery looks and sounds like – as well as scenarios that do not qualify as package delivery, such as a mail carrier leaving an envelope in a mailbox.
Keys to Doing It Right
A business needs to manage many moving parts to act carefully and mindfully, such as:
- Respecting privacy in each country where the business operates and its customers live. This means following legal requirements as well as practical considerations. For instance, businesses need to define boundaries for the use of cameras, agree on policies for how visual images can be stored, and decide on how to notify customers that cameras are being used to safeguard a physical location.
- Being mindful. It’s important that businesses be careful to apply the principles of Mindful AI when developing computer vision, such as relying on a diverse team of development professionals to safeguard against bias creeping into the process of developing data for AI. Applied without a mindful approach, behavioral data capture can create ethical issues, such as profiling shoppers based on their appearance instead of their behavior. In addition, a diverse team is needed to be inclusive. Professionals with different backgrounds can help train AI to account for human behaviors in context of different cultures and contexts.
- Using technology to scale the training. One of the biggest impediments to any AI application is processing all the data needed to train AI. Businesses need a common platform to collect multiple data samples, manage that data, and store it. In addition, data collection requires effective management of the workflow, from scheduling projects to assigning deadlines. (Centific provides this through our OneForma platform.)
Centific applies those principles in our own work in this field.
A Solution from Centific
Behavioral data capture is one of the tools used by Centific in our Scout solution. Scout is a comprehensive platform that puts retailers in control of their stores, empowering them to:
- Reduce shrink.
- Prevent stock-outs.
- Improve customer satisfaction.
- Deliver financial value to stakeholders.
At the heart of Scout is behavior anomaly detection. An integrated insights platform provides personalized and prescriptive analytics in real-time, intuitively alerting a store’s team to events before they escalate.
Scout's human-in-the-loop foundation mitigates bias through comprehensive training data sets powered and annotated in our OneForma platform,leveraging Centific's global team of risk mitigation experts for real-time situational analysis, decreasing false positives. As Scout's knowledge of patterns grows, they are shared with users through the exclusive and secure pattern recognition network.
Whether it's understanding a particular customer's behavior, detecting fraud at self-checkouts, or identifying on-premises hazards, Scout empowers retailers to act with confidence in real-time. To learn more, contact Centifc.