How the Right LLM Can Empower Data Insights in Pharma
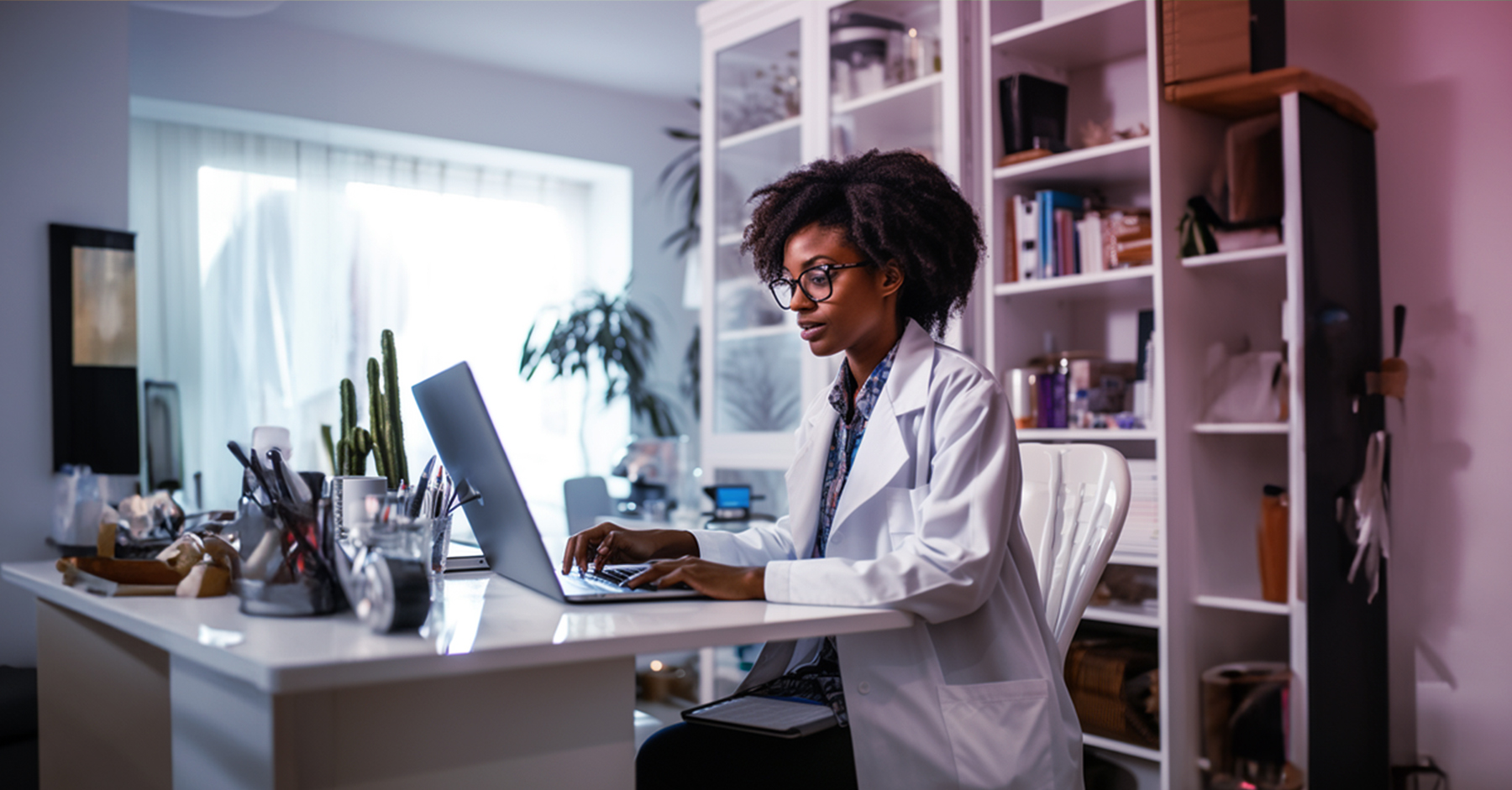
How can large language models help a multi-billion-dollar business develop products faster at a global level? Read on to learn how Centific is applying our Honeybee AI toolkit to improve the way a leading pharmaceutical business develops essential medicines across several countries.
About Our Client
Our client is a major pharmaceutical and biotechnology company. The company focuses on the discovery, development, and commercialization of prescription medicines in oncology, rare diseases, and biopharmaceuticals, including cardiovascular, renal and metabolism, and respiratory and immunology. Its medicines are used by millions of patients worldwide across 100 countries.
The Business Problem
The company asked Centific to help tackle an enormous challenge: how might we help manage the increasingly vast lakes of data required to develop its portfolio of drugs? A business that corrals data more efficiently can develop much-needed treatments faster for various global populations. AI can help, but it must be applied in a responsible way.
Digging Deeper into the Challenge
The safe development of any prescription medicine requires a pharmaceutical business to organize and interpret enormous amounts of highly complex and diverse data. This data includes details about drugs, their chemical properties, their impacts on human physiology, their interactions with other drugs, patient responses, and much more.
Each of these data points can be conceptualized as part of a larger ontology -- a formal representation of knowledge about a particular domain, such as drug discovery or clinical trials. For instance, ontologies can be used to represent clinical trial data, such as patient demographics, medical history, and treatment outcomes. This data can then be used to identify patterns and trends, and to make better decisions about the design and conduct of clinical trials.
Our client asked Centific to find a better way to organize and annotate data in order to develop treatments faster and more broadly across its 100 markets. Speed of product development was important – but so was scale. Each drug developed by the company must be effective for every possible segment of the population that the company serves, and of course myriad human factors influence the efficacy of any treatment, such as the age and gender of a potential patient.
The Solution: Honeybee
Centific saw a solution: large language models (LLMs) could improve the workflow required to manage functions ranging from data interpretation to knowledge discovery.
But Centific ruled out commercial off-the-shelf LLM-based applications such as ChatGPT or Bard. Third-party LLMs do not guarantee the transparency of the data training and processing pipelines. Information such as how the data is used, stored, or used for retraining is often unclear – which is not ideal for usage in highly secure and regulated industries such healthcare. Personal health information that is transmitted, stored, or accessed electronically also falls under HIPAA regulatory standards (and is known as electronic protected health information, or ePHI).
In addition, LLMs must be trained carefully so that they are applied in a responsible manner. For instance, any LLM must respect patient privacy when digging through patient data. Instead of going with a commercial tool, Centific has applied our own: Honeybee, our framework that finetunes LLMs to make work processes faster and more effective. For our client, Centific is using Honeybee with an open-sourced LLM hosted locally. Training data adapted to each healthcare use case to ensure a secure and transparent process. To ensure that AI is applied responsibly, Centific is relying on a human-in-the-loop approach. Life sciences experts perform crucial roles such as evaluating the model, thus flagging issues such as inaccuracies and biases.
In order to provide accurate results for each use case at scale, Honeybee also relies on an “LLM Farm” approach, where multiple finetuned LLMs are queried for the same prompt – and the one with higher confidence is taken as the end result for the customer. This makes Honeybee the best companion to leverage LLMs for a variety of use cases, in multiple industries.
Honeybee in Action
With Honeybee, our client can more effectively and quickly:
- Understand different data types and sources, ranging from structured data in databases to unstructured data like scientific literature or clinical notes. It can integrate this information into a coherent framework.
- Analyze large data sets and discover patterns or trends that might not be apparent to human researchers. Honeybee can annotate documents (such as medical records) and show insights based on information and ontologies that have been fed to the model. Furthermore, Honeybee uses those insights to annotate new documents and gather insights from data lake. Consequently, this information can be used to make decisions faster, including helping medical personnel in making diagnoses more quickly.
- Do more targeted and accurate search. For instance, a researcher can use the LLM to find all drugs that target a specific pathway and have been effective in a particular type of cancer. The LLM can then return not only a list of drugs but also an explanation of their mechanisms of action and key supporting evidence.
- Maintain and update an ontology by scanning new research and industry updates, interpreting their content, and then automatically updating the ontology accordingly. This helps ensure that the ontology stays current with the latest scientific knowledge.
Honeybee manages the substantial work required for AI training, system design, and validation. Plus, given the sensitive and critical nature of pharmaceutical data, Honeybee ensures that the data annotation is done with a keen eye for accuracy, privacy, and security.
Results
The collaboration is expected to deliver these results:
- Increased efficiency: a significant reduction in manual annotation time by up to 70%. This enables more effective data-driven decisions.
- Improved accuracy: an improvement in the precision of annotations by up to 40%. This ensures AI models are trained with high-quality, relevant data.
- Scalability: successful management of large volumes of healthcare data to deliver insights and drive innovation across the entire AI application lifecycle.
- Data security: the preservation of sensitive healthcare data by employing strict data privacy measures. As noted, the LLM employed is hosted entirely locally. This means data resides on an infrastructure that is controlled directly by the client. Data used for training is strictly controlled, respecting high levels of security and health regulations – especially when compared with other cloud-based commercial solutions.
By making data-driven decisions more efficient and accurate, our client will ultimately benefit from developing high-quality medicines to market faster and responsibly. To learn how we can help you do the same, contact us.